“Generative Pre-trained Transformer,” or GPT for short, is a groundbreaking technology created by OpenAI that powers several sophisticated language models such as Chat GPT. It has transformed the field of natural language processing (NLP) by allowing machines to produce text with human-like fluency and coherence. However, in the case of Chat GPT specifically, what does this acronym mean and how exactly does it function?
The Evolution of GPT Models
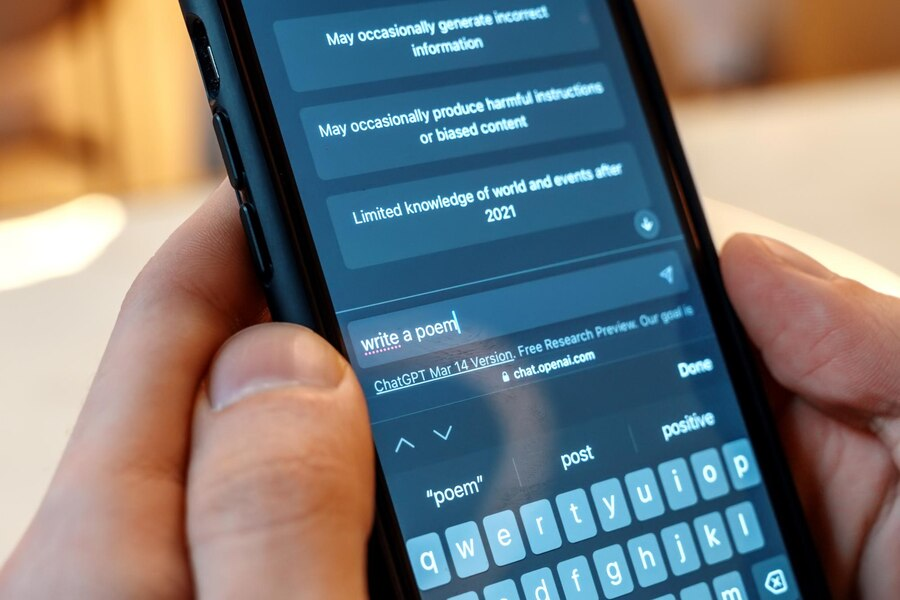
GPT has undergone significant advancements since its inception. Here’s a brief timeline:
Model | Release Year | Parameters | Notable Features |
---|---|---|---|
GPT-1 | 2018 | 117 million | First introduction of the transformer model |
GPT-2 | 2019 | 1.5 billion | Significant increase in parameters, improved coherence |
GPT-3 | 2020 | 175 billion | Massive leap in parameters, enhanced understanding |
GPT-3.5 | 2022 | 175 billion+ tweaks | Improved efficiency and fine-tuning |
GPT-4 | 2023 | Over 500 billion | Multimodal capabilities, better context handling |
What Does GPT Stand For in Chat GPT?
GPT stands for Generative Pre-trained Transformer, reflecting its three core components:
Generative
ChatGPT has generative abilities at its heart, which enable it to produce text from given input. This capacity for generating content gives ChatGPT the ability to participate in conversations, respond to inquiries and create various forms of content.
- Natural Language Generation: The ChatGPT’s generative model emulates human-like replies by generating text in real time, influenced by the input it obtains;
- Contextual Understanding: With its ability to generate, ChatGPT can modify its responses according to diverse conversational situations while ensuring consistency and pertinence;
- Creative Writing: GPT’s versatility goes beyond mere text generation as it can perform creative tasks like poetry creation, story generation and content synthesis with its generative nature.
Pre-trained
ChatGPT undergoes extensive pre-training on massive amounts of text data, allowing it to gain a thorough grasp of language before being fine-tuned for particular tasks. This prior training equips the model with comprehensive knowledge and enables it to produce articulate responses across diverse topics and domains.
- Foundation of Knowledge: A strong knowledge base is built by ChatGPT during pre-training as it acquires grammar, syntax, semantics and context from a wide range of textual datasets;
- Adaptability: The adaptability of the pre-trained model enables it to adjust to varying conversational contexts and user prompts, utilizing its knowledge in language comprehension to produce pertinent replies;
- Specialization through Fine-tuning: With fine-tuning, ChatGPT can specialize in particular tasks or domains and improve its effectiveness and suitability for specific applications. Pre-training grants a general knowledge base, but specialization is accomplished through the process of fine-tuning.
Transformer
Utilizing a transformer architecture known for efficiently managing sequential data such as text, ChatGPT effectively captures long-range dependencies and generates coherent responses thanks to its self-attention mechanisms.
- Efficient Processing: The use of Transformer architecture enables ChatGPT to efficiently process input text by simultaneously attending to various sections of the sequence;
- Contextual Understanding: By utilizing self-attention mechanisms, ChatGPT can evaluate the relevance of individual words or tokens within the given input, ultimately enhancing its comprehension of contextual and semantic factors;
- Real-time Applications: ChatGPT’s transformer architecture enables parallel computation, making it suitable for real-time applications like chatbots and conversational agents. This guarantees accurate and timely responses.
Understanding GPT-3.5
GPT-3.5, a progression from GPT-3, integrates several adjustments and enhancements to elevate its performance. Despite maintaining the equivalent parameter count as GPT-3,GPT 3.5 presents:
Better Fine-tuning Capabilities
With enhanced fine-tuning capabilities, GPT-3.5 allows for more effective tailoring of its behavior to specific domains or tasks. This advancement enables finer adjustments to the model’s parameters and thus improves performance on specialized tasks.
- Fine-tuning Flexibility: GPT-3.5 enhances flexibility in fine-tuning the model for specific applications, enabling users to optimize performance by adjusting parameters like learning rate, batch size and optimization strategies tailored for their use case;
- Task-specific Optimization: By utilizing the superior fine-tuning capabilities of GPT-3.5, individuals can tailor the model’s effectiveness to particular tasks such as text categorization, analyzing sentiments or addressing queries. Through adeptly modifying it according to task-specific datasets, users are able to improve its precision and overall performance.
Improved Contextual Understanding
Compared to its predecessor, GPT-3.5 exhibits upgraded contextual comprehension which enhances the accuracy and relevance of responses in natural language processing tasks. This development further strengthens the model’s capacity to grasp intricate aspects of language and produce coherent textual outputs.
- Contextual Adaptation: GPT-3.5 showcases its proficiency in adjusting to various conversational settings, which empowers it to create answers that are better attuned with the corresponding context. The algorithm can exploit contextual hints to produce responses that are more appropriate and seamless;
- Semantic Understanding: By enhancing its contextual comprehension, GPT-3.5 has elevated its semantic understanding of text to a more efficient level. This advancement empowers the model to perceive and express subtle intricacies in language with greater precision, thereby producing responses that demonstrate a profound knowledge of the given input.
Enhanced Efficiency in Generating Coherent Responses
The improved GPT-3.5 demonstrates heightened proficiency in generating logical responses, resulting in faster and more precise text production. These advancements optimize the model’s functionality for real-time implementations as well as demanding tasks of extensive scale pertaining to written communication.
- Faster Response Generation: GPT-3.5 enables faster response generation than its previous version, leading to reduced latency and improved responsiveness in conversational applications. This upgrade results in smoother and more authentic interactions with users;
- Reduced Repetition and Incoherence: By boosting its efficacy, GPT-3.5 lessens the occurrence of repetition and incoherence within generated text, resulting in more coherent and fluent output. This technology enables lengthier passages to be produced with minimal errors which ultimately elevates the standard of generated content.
GPT-4: The Next Leap
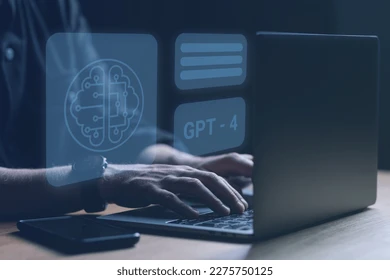
GPT-4, the latest iteration, brings substantial advancements:
Increased Parameters
With over 500 billion parameters, GPT-4 represents a considerable advancement in comparison to its forerunners. Such an increase bestows the model with greater intricacy and enhances its capacity for generating more sophisticated text while also improving contextual comprehension, ultimately leading to contextually relevant responses.
- Parameter Count: The Parameter Count of GPT-4 has exceeded previous models, with more than 500 billion parameters. This enhances its capabilities to grasp intricate linguistic subtleties and contextual intricacies;
- Nuanced Text Generation: Text generation has become more nuanced with GPT-4 thanks to its increased parameters. This enhancement allows for the creation of text that is both complex and profound, resulting in responses that are contextually appropriate across various tasks and domains.
Multimodal Capabilities
GPT-4 introduces multimodal capabilities, allowing it to process both text and images seamlessly. This enhancement enables the model to incorporate visual information into its understanding and generation of text, opening up new possibilities for applications in fields such as image captioning, visual storytelling, and more.
- Text-Image Fusion: GPT-4 can effectively integrate textual and visual information, enhancing its comprehension and generation abilities in tasks involving both modalities;
- Image Understanding: With multimodal capabilities, GPT-4 gains the ability to understand and interpret visual content, enabling it to generate text descriptions, captions, and responses based on images.
Enhanced Context Handling
GPT-4 demonstrates improved capabilities in handling complex instructions and maintaining context over longer interactions. This enhancement enables the model to understand and respond to multi-turn conversations, long-form texts, and intricate instructions more effectively.
- Complex Instruction Understanding: GPT-4 excels in parsing and understanding complex instructions, enabling it to generate responses that accurately reflect the given instructions;
- Long-Term Context Maintenance: With enhanced context handling, GPT-4 can maintain context over longer interactions, ensuring coherence and relevance in multi-turn conversations and extended texts.
How GPT Works: A Technical Overview
Understanding what GPT stands for in Chat GPT requires a dive into its technical workings:
Transformer Architecture
At the core of GPT models lies the transformer architecture, which serves as the backbone for their operations. Transformers employ self-attention mechanisms to process input sequences and generate output sequences. This architecture excels in handling sequential data, making it well-suited for natural language processing tasks.
- Self-Attention Mechanisms: Transformers utilize self-attention mechanisms to weigh the importance of different words in a sentence. By attending to various parts of the input sequence simultaneously, transformers can capture long-range dependencies and contextual information effectively;
- Parallel Computation: The parallel nature of self-attention mechanisms allows transformers to process input sequences efficiently, enabling faster training and inference times;
- Multi-Head Attention: Transformers often incorporate multi-head attention mechanisms, which allow them to focus on different aspects of the input sequence concurrently. This enhances the model’s ability to capture diverse features and nuances in the input data.
Pre-training and Fine-tuning
GPT models undergo a two-stage training process: pre-training and fine-tuning. During the pre-training phase, the model is exposed to vast amounts of diverse text data from the internet. This pre-training enables the model to learn the intricacies of language, including grammar, syntax, semantics, and context.
- Data Pre-processing: Before pre-training, the input text data undergoes pre-processing, including tokenization, padding, and encoding, to prepare it for input into the model;
- Masked Language Modeling: In pre-training, GPT models often employ masked language modeling objectives, where certain tokens in the input sequence are masked, and the model is trained to predict the masked tokens based on surrounding context;
- Fine-tuning on Specific Tasks: After pre-training, GPT models are fine-tuned on specific datasets relevant to the desired task. Fine-tuning involves adjusting the model’s parameters to optimize performance on the target task while retaining the knowledge gained during pre-training.
Applications of GPT
GPT’s versatility makes it suitable for various applications:
Chatbots
GPT powers conversational agents and chatbots, providing human-like interactions and personalized responses to users’ queries and prompts. By leveraging its generative capabilities and contextual understanding, GPT enables engaging and effective communication with users in various contexts.
- Human-like Interactions: GPT facilitates natural and seamless interactions between users and chatbots, enhancing user experience and satisfaction;
- Personalized Responses: GPT can generate responses tailored to individual users’ preferences and needs, creating a more personalized and engaging conversational experience;
- 24/7 Availability: Chatbots powered by GPT can operate around the clock, providing instant assistance and support to users whenever they need it.
Content Creation
GPT excels in generating diverse forms of content, including articles, summaries, and creative writing pieces. By leveraging its generative capabilities and broad understanding of language, GPT can produce high-quality and coherent content on a wide range of topics.
- Article Generation: GPT can generate informative and well-structured articles on various subjects, providing valuable content for websites, blogs, and publications;
- Summarization: GPT can summarize lengthy texts or documents, distilling key information and insights into concise summaries;
- Creative Writing: GPT can assist writers in generating ideas, plots, characters, and dialogue for stories, scripts, or poetry, sparking creativity and supporting the creative process.
Customer Support
GPT automates responses to common customer queries, streamlining customer support processes and improving efficiency. By understanding and generating contextually relevant responses, GPT enables businesses to provide timely assistance to customers and resolve issues effectively.
- Automated Responses: GPT can generate responses to frequently asked questions or common customer inquiries, reducing the workload on human customer support agents;
- Efficient Resolution: GPT’s ability to understand and generate accurate responses allows businesses to address customer queries and concerns promptly, leading to higher customer satisfaction;
- Scalability: Automated customer support powered by GPT can scale to handle large volumes of customer inquiries efficiently, ensuring consistent and reliable support services.
Language Translation
GPT facilitates language translation tasks by accurately translating text between different languages. Leveraging its contextual understanding and multilingual capabilities, GPT produces high-quality translations that preserve the meaning and nuances of the original text.
- Accurate Translation: GPT generates translations with high accuracy, capturing the meaning and context of the source text to produce natural and fluent translations;
- Multilingual Support: GPT supports translation between multiple language pairs, allowing users to translate text across different languages with ease;
- Contextual Preservation: GPT preserves the context and nuances of the original text during translation, ensuring that the translated text accurately reflects the intended meaning and tone.
Benefits of Using GPT in Chat GPT
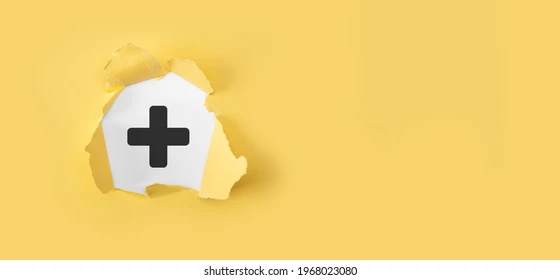
Utilizing GPT in chat applications offers several advantages:
Natural Conversations
One of the primary benefits of using GPT in Chat GPT is its ability to generate responses that closely mimic human conversation patterns. By leveraging its generative capabilities and contextual understanding, GPT can produce responses that are natural, coherent, and contextually relevant, creating a more engaging and lifelike conversational experience for users.
- Contextual Understanding: GPT excels in understanding the context of user queries and generating responses that align with the given context, leading to more meaningful and relevant conversations;
- Human-like Responses: GPT generates responses that exhibit human-like conversational patterns, including appropriate tone, style, and language use, enhancing the overall conversational experience for users;
- Engagement: By simulating natural conversations, GPT encourages users to engage more actively in conversations, leading to longer interactions and increased user satisfaction.
Scalability
GPT offers scalability, allowing Chat GPT to handle large volumes of interactions simultaneously without compromising performance or responsiveness. This scalability is particularly beneficial for chat applications that experience high traffic or require real-time interactions with multiple users.
- High Throughput: GPT’s efficient architecture enables Chat GPT to process a large number of user interactions concurrently, ensuring smooth and uninterrupted communication even during peak usage periods;
- Real-time Interaction: With its ability to scale, Chat GPT can provide real-time responses to users, minimizing latency and enhancing the user experience;
- Flexibility: GPT’s scalability makes it suitable for deployment in a variety of environments, from small-scale chat applications to large-scale platforms with millions of users.
Cost Efficiency
Integrating GPT into Chat GPT can lead to cost efficiency by reducing the need for extensive human intervention in customer support and other communication processes. By automating responses to common queries and tasks, Chat GPT powered by GPT can streamline operations and reduce overhead costs associated with human labor.
- Automation: GPT automates responses to common queries and tasks, freeing up human agents to focus on more complex or specialized inquiries, improving productivity and efficiency;
- Reduced Workload: By handling routine interactions, Chat GPT powered by GPT reduces the workload on human agents, enabling them to handle higher-value tasks and improve overall service quality;
- 24/7 Availability: GPT-powered Chat GPT can operate around the clock, providing continuous support to users without the need for human agents to be available at all times, further enhancing cost efficiency.
Challenges and Limitations of GPT
Despite its capabilities, GPT models face certain challenges:
Bias
A significant challenge faced by GPT models is the presence of bias in the data on which they are pre-trained. Since GPT models are typically pre-trained on vast amounts of internet text, they may inadvertently learn and perpetuate societal biases present in the data. This bias can manifest in various forms, including gender, racial, cultural, or ideological biases, and may lead to biased or unfair outputs generated by the model.
- Unintended Biases: GPT models may exhibit biases in their responses, reflecting and reinforcing societal biases present in the pre-training data;
- Mitigation Strategies: Addressing bias in GPT models requires careful data curation, bias detection techniques, and bias mitigation strategies during both pre-training and fine-tuning stages;
- Ethical Considerations: Addressing bias in GPT models is essential from an ethical standpoint to ensure fairness, equity, and inclusivity in their applications across different domains and contexts.
Resource Intensive
Training and running large GPT models can be resource-intensive, requiring significant computational resources in terms of processing power, memory, and storage. The sheer scale and complexity of large GPT models necessitate powerful hardware infrastructure and substantial computational resources, which may pose challenges for organizations with limited resources or budgets.
- Computational Requirements: Training large GPT models involves processing vast amounts of data and performing complex computations, which can strain computational resources and infrastructure;
- Cost Considerations: The resource-intensive nature of training and running large GPT models can result in high costs associated with hardware, cloud computing services, and energy consumption;
- Scalability Challenges: Scaling up GPT models to larger sizes or deploying them in production environments may require additional investments in infrastructure and optimization to ensure efficient performance and scalability.
Contextual Understanding
Maintaining context over very long conversations presents a challenge for GPT models, particularly in multi-turn dialogues or interactions that span extended periods. While GPT models demonstrate impressive contextual understanding, they may struggle to maintain coherence and relevance over extended conversations, leading to potential misunderstandings or inconsistencies in responses.
- Long-term Context: GPT models may have difficulty retaining and integrating context from earlier parts of a conversation into later responses, leading to breakdowns in coherence and relevance;
- Context Switching: Managing multiple topics or shifting contexts within a conversation can challenge GPT models’ ability to maintain focus and relevance over time;
- Mitigation Strategies: Techniques such as memory augmentation, context windowing, or hierarchical modeling can help GPT models better manage long-term context and improve their performance in extended interactions.
Future of GPT
The future of GPT looks promising, with ongoing research focusing on:
Reducing Bias
Addressing bias in generated text is a critical area of research for the future of GPT models. By implementing techniques to minimize biases in the training data and model architecture, researchers aim to produce more fair, equitable, and unbiased text generation outputs.
- Bias Detection: Developing algorithms and methods to detect and quantify biases present in training data and generated text, enabling researchers to identify and mitigate potential biases effectively;
- Bias Mitigation Techniques: Implementing strategies such as data augmentation, debiasing algorithms, and fairness constraints to minimize biases in GPT models and ensure more balanced and inclusive text generation;
- Ethical Considerations: Incorporating ethical considerations into the design and development of GPT models, including transparency, accountability, and fairness, to promote responsible and ethical use of AI technologies.
Increasing Efficiency
Improving the efficiency of GPT models is another crucial area of research for the future, aiming to reduce computational requirements and enhance scalability. By developing more efficient architectures and optimization techniques, researchers seek to make GPT models more accessible and sustainable for a broader range of applications.
- Model Compression: Exploring techniques such as pruning, quantization, and knowledge distillation to compress and simplify GPT models, reducing memory footprint and computational overhead while preserving performance;
- Hardware Acceleration: Leveraging specialized hardware accelerators, such as GPUs, TPUs, or custom AI chips, to accelerate the training and inference processes of GPT models, improving efficiency and reducing energy consumption;
- Algorithmic Improvements: Investigating novel algorithms and optimization strategies to streamline training and inference pipelines, maximizing computational efficiency and resource utilization in GPT models.
Enhancing Multimodal Capabilities
Expanding beyond text and images, enhancing GPT models’ multimodal capabilities to include audio and video processing represents an exciting frontier for future research. By integrating multiple modalities of data, researchers aim to create more versatile and expressive AI systems capable of understanding and generating diverse forms of content.
- Audio Processing: Developing techniques for incorporating audio signals, such as speech or sound, into GPT models, enabling them to understand and generate spoken language or audio-based content;
- Video Processing: Extending GPT models to process video data, including visual information and temporal dynamics, to enable tasks such as video captioning, scene understanding, and video generation;
- Multimodal Fusion: Investigating methods for fusing information from different modalities, such as text, images, audio, and video, to create comprehensive representations and generate rich, multimodal outputs.
Conclusion
Understanding what GPT stands for in Chat GPT reveals the remarkable progress in natural language processing technology. From GPT-1 to the latest GPT-4, each iteration brings enhanced capabilities, making AI interactions more natural and efficient. As research continues, we can expect even more sophisticated and versatile GPT models in the future.
This comprehensive guide aims to provide a thorough understanding of what GPT stands for in Chat GPT, shedding light on the technology’s evolution, applications, benefits, challenges, and future directions. With GPT models continually advancing, the possibilities for their use in various domains are expanding, promising exciting developments in the field of AI and machine learning.
FAQ
GPT stands for Generative Pre-trained Transformer.
GPT-3.5 includes various improvements and tweaks over GPT-3, enhancing efficiency and contextual understanding.
GPT-4 offers increased parameters, multimodal capabilities, and enhanced context handling.
GPT is used in chatbots, content creation, customer support, and language translation.
Key challenges include bias in generated text, high computational requirements, and difficulties in maintaining context over long conversations.